The Army has built the largest facial recognition database of thermal images
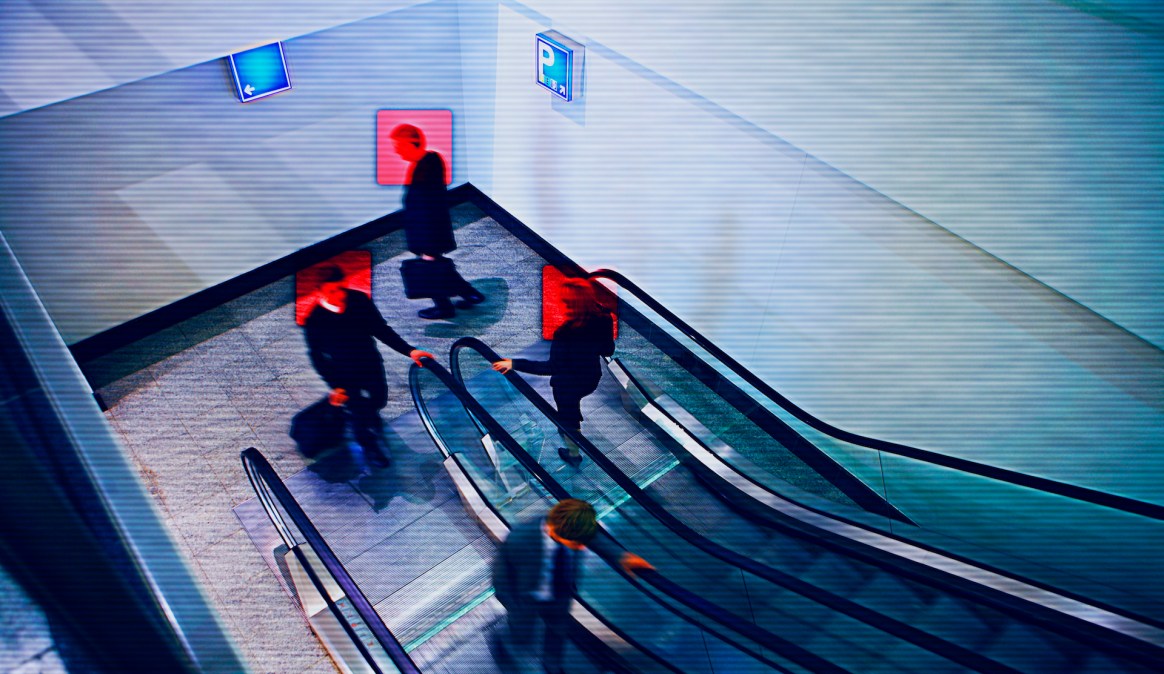
The Army has built the largest public dataset in the world of thermal images of faces — a major step in expanding the technology’s ability to identify people in the cover of darkness using artificial intelligence.
The service announced the creation of the Army Research Laboratory Visible-Thermal Face Dataset in a recent research paper. The dataset has more than half-a-million images of 395 subjects.
“This dataset is, to the best of our knowledge, the largest thermal face dataset publicly available for scientific research to date,” stated a paper from the Army Research Lab, which partners with West Virginia University, Booz Allen Hamilton, Johns Hopkins and the University of Nebraska-Lincoln in the project.
Such a dataset is important to the Army and other organizations that run 24/7 operations and don’t always have the benefit of sunlight when identifying a subject. The Army’s work could make facial recognition technology even more ubiquitous down the line for federal agencies, giving them the ability to work in the dark and the light.
Facial recognition is in the news of late for its use in identifying pro-Trump rioters who stormed the Capitol last week. There is still a “large gap” between the type of facial recognition technology used for that type of identification and the thermal image identification the Army hopes to improve upon, as thermal images have lower definition and quality. But, there have been recent advances in technology in the thermal cameras themselves, which can now render higher quality images with more defining features.
With the dataset of images, now comes the heavy lifting of artificial intelligence where models need to be trained on the data and adjusted for accuracy. The use of such facial recognition is a controversial topic due to its trained bias and lack of accuracy — especially on people of color and women — and privacy concerns.
The dataset is not perfect nor will it immediately lead to the night-and-day ability for facial recognition to identify people. The paper indicates that initial algorithms used with the dataset failed when subjects wore glasses or their face was “off-pose” from images in the training data. It’s also relatively small in scale. Other successful databases used to recognize faces in normal images tend to be much larger, such as Clearview AI’s. which claims to have billions of scraped photos in its dataset.